微信扫码
添加专属顾问
我要投稿
探索高效处理扫描版PDF的革命性解决方案,郭震带你体验智能体源码的神奇魅力! 核心内容: 1. 扫描版PDF到Word文档的高效转换方案 2. 基于Qwen2.5-VL-7B模型的多模态理解与自动处理 3. 利用远程GPU算力,实现快速批量处理
nvidia-smi --list-gpus
安装torch,transformers等,直接复制下面两行命令执行:
pip install torch torchvision --index-url https://download.pytorch.org/whl/cu121pip install transformers safetensors
如果要处理视频或多模态任务,再安装opencv-python:
pip install opencv-python
pip install accelerate
from transformers import Qwen2_5_VLForConditionalGeneration, AutoTokenizer, AutoProcessorfrom qwen_vl_utils import process_vision_info# default: Load the model on the available device(s)model = Qwen2_5_VLForConditionalGeneration.from_pretrained( "Qwen/Qwen2.5-VL-7B-Instruct", torch_dtype="auto", device_map="auto")# We recommend enabling flash_attention_2 for better acceleration and memory saving, especially in multi-image and video scenarios.# model = Qwen2_5_VLForConditionalGeneration.from_pretrained(# "Qwen/Qwen2.5-VL-7B-Instruct",# torch_dtype=torch.bfloat16,# attn_implementation="flash_attention_2",# device_map="auto",# )# default processerprocessor = AutoProcessor.from_pretrained("Qwen/Qwen2.5-VL-7B-Instruct")# The default range for the number of visual tokens per image in the model is 4-16384.# You can set min_pixels and max_pixels according to your needs, such as a token range of 256-1280, to balance performance and cost.# min_pixels = 256*28*28# max_pixels = 1280*28*28# processor = AutoProcessor.from_pretrained("Qwen/Qwen2.5-VL-7B-Instruct", min_pixels=min_pixels, max_pixels=max_pixels)messages = [ { "role": "user", "content": [ { "type": "image", "image": "https://qianwen-res.oss-cn-beijing.aliyuncs.com/Qwen-VL/assets/demo.jpeg", }, {"type": "text", "text": "请用中文描述这张图片的内容。"}, ], }]# Preparation for inferencetext = processor.apply_chat_template( messages, tokenize=False, add_generation_prompt=True)image_inputs, video_inputs = process_vision_info(messages)inputs = processor( text=[text], images=image_inputs, videos=video_inputs, padding=True, return_tensors="pt",)inputs = inputs.to("cuda")# Inference: Generation of the outputgenerated_ids = model.generate(**inputs, max_new_tokens=128)generated_ids_trimmed = [ out_ids[len(in_ids) :] for in_ids, out_ids in zip(inputs.input_ids, generated_ids)]output_text = processor.batch_decode( generated_ids_trimmed, skip_special_tokens=True, clean_up_tokenization_spaces=False)print(output_text)
因为本地还没有下载过"Qwen2.5-VL-7B"
模型,所以 transformers
框架自动去 Huggingface 下载模型的全部权重文件。model.safetensors.index.json 是模型的索引文件。model-00001-of-00005.safetensors 等这几个是模型的权重分片(大模型通常被切成多个小块,分片下载)。
咱们默认输入如下这个图片,让它理解下这个图的信息:
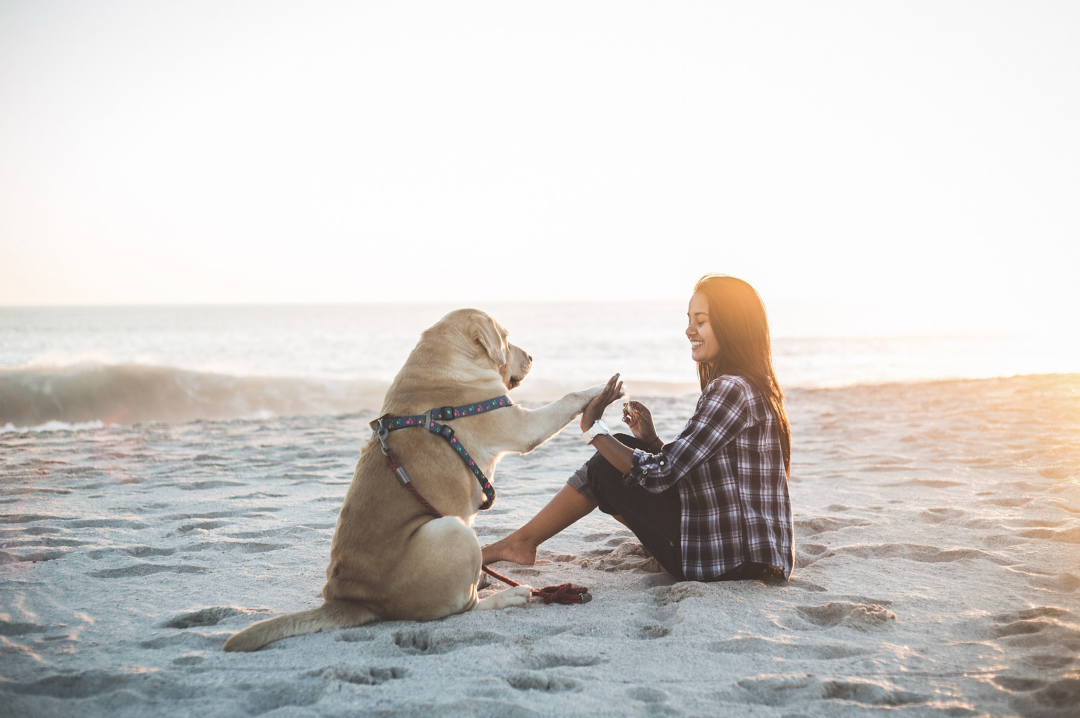
![]()
![]()
返回英文了:
咱们修改下代码这里,提示词告诉它使用中文描述:
下面是中文返回结果,如下图所示:
以上描述结果,大家觉得如何,有没有觉得很精准呀。
4 扫描版PDF开始转Word
上传一个300多页的pdf文件到服务器,使用scp命令:
scp -P 41277 ocr_test.pdf root@你的服务器名字(来源文章一开始复制的登录地址)
提示输入密码后,输入文章一开始登录地方的密码,如下图所示:
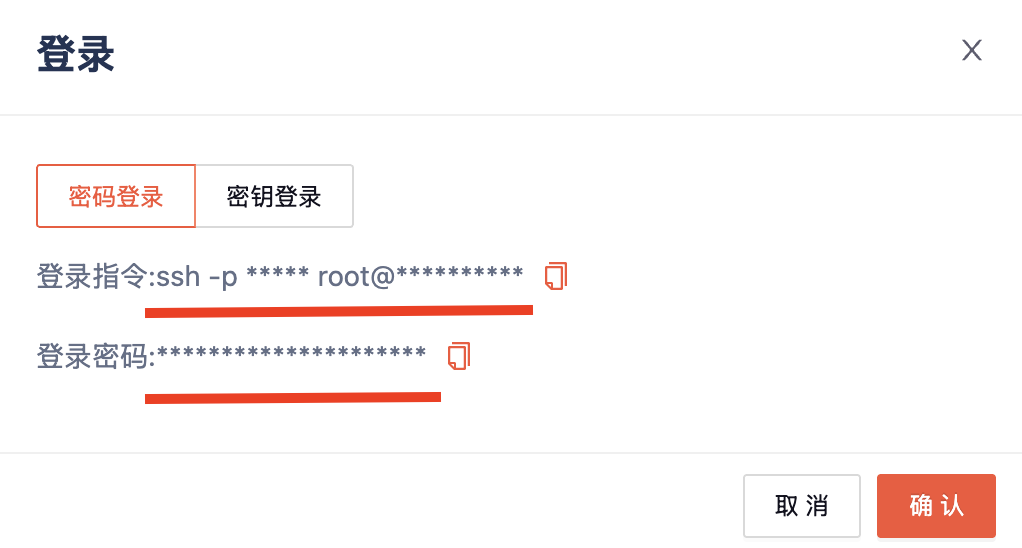
![]()
![]()
再安装PDF转Image的包:
pip install pdf2image python-docx
sudo apt updatesudo apt install poppler-utils
53AI,企业落地大模型首选服务商
产品:场景落地咨询+大模型应用平台+行业解决方案
承诺:免费场景POC验证,效果验证后签署服务协议。零风险落地应用大模型,已交付160+中大型企业
2025-06-11
AI提效99.5%!英国政府联手 Gemini,破解城市规划审批困局
2025-06-10
多模态 RAG VS 传统文本 RAG ,到底效果如何,从应用视角来测试下
2025-06-10
实战复盘 | 基于视觉模型的多模态 RAG 系统,我们踩过的坑与收获 (项目已开源)
2025-06-05
多模态模型在RagFlow中的应用
2025-06-04
清华首创多模态+知识图谱+RAG,问答精准度超 94%
2025-05-30
Deepseek 多模态来解析图片,结合上下文分析pdf文档
2025-05-28
Lovart再次证明:AI不是卖工具而是卖成果
2025-05-27
Dolphin-API:字节Dolphin多模态文档解析模型API化全攻略
2025-05-14
2025-03-26
2025-03-21
2025-04-27
2025-05-16
2025-05-08
2025-04-28
2025-04-05
2025-05-13
2025-05-15